1. 混合交通环境下交叉路口信号灯-车辆协同控制方法
交叉路口是城市中的常见场景,也是影响道路交通效率和车辆燃油经济性的重要因素。当交通拥堵发生时,交叉路口信号灯会导致车辆频繁的启停及怠速,带来了车辆燃油经济性的下降,而常见固定配时的信号灯控制方法难以解决的交通拥堵问题。智能网联汽车的出现为进一步的交通优化提供了可能,一方面,智能网联汽车可以得到信号灯配时等交通环境信息,对自车的行驶轨迹进行优化;另一方面,智能网联汽车可以获取周围其他车辆的信息,通过对自车的控制改善区域交通效率。然而,智能网联汽车的普及仍需数十年的时间,现有对人工驾驶车辆与网联自动驾驶车辆共存的车辆协同控制算法研究未能充分考虑驾驶人驾驶车辆的信息。研究针对混合交通环境,提出了智能网联车辆队列形成方法及分层优化信号灯控制方法,可以在不同市场渗透率下对交通效率和燃油经济性进行优化。
为考虑人工驾驶车辆的行驶情况,研究提出了混合交通下的混合队列形成方法,通过将智能网联汽车与驾驶人驾驶车辆组合成为“ 1+N ”的队列模式,使智能网联汽车主动引导后续驾驶人驾驶车辆通过交叉路口。基于系统分析,证明了保证混合队列的稳定性、可控性的充要条件,为混合队列的控制提供了理论基础。
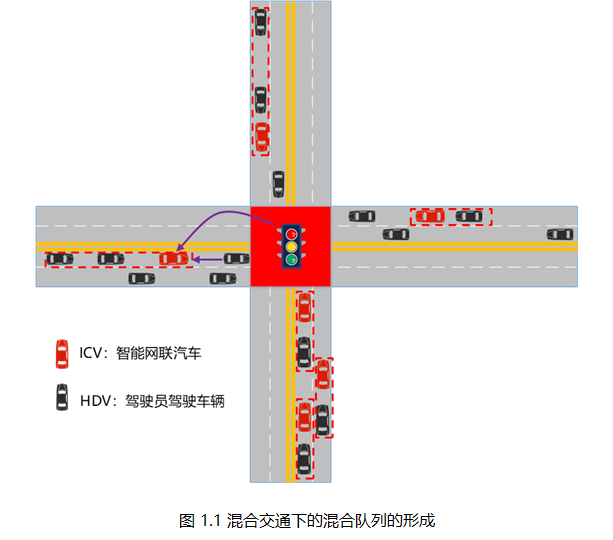
通过对驾驶人驾驶车辆跟车模型的研究,提出了固定配时下最优跟车间距和最大可通行车辆数目的确定方法。对固定长度的队列驶入交叉路口的过程,提出了混合车辆队列最优控制模型,使用基于伪谱法的最优控制方法进行轨迹规划,实现了对燃油经济性的优化。
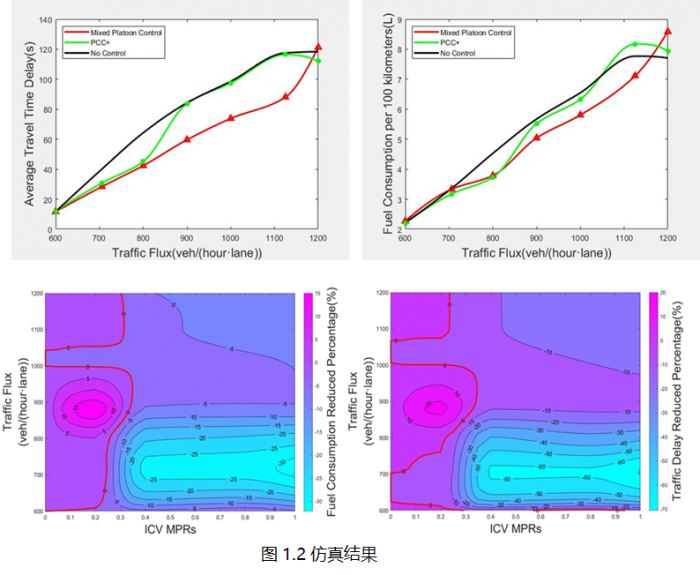
驾驶人驾驶车辆通过交叉路口时需信号灯引导,因此针对提出的混合交通队列模型,设计了混合交通下的预约式信号灯控制方法。扩展了常见的“先到先得式”智能网联单车优先级分配模型,设计了混合交通下考虑队列长度的综合优先级模型分配方法,实现了八相位下的信号灯时间动态分配,可以综合优化整体交叉路口的通行效率。搭建了基于微观交通流模型的仿真分析平台,对混合队列算法有效性进行了验证。
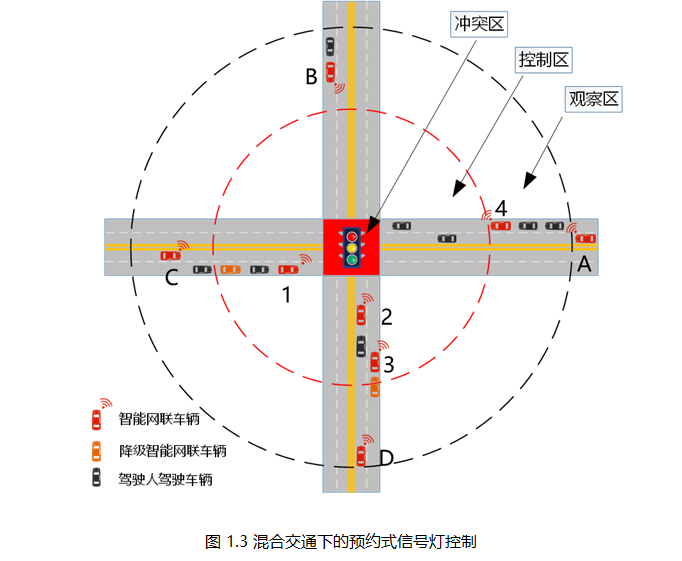
研究骨干:陈超义
2. 非理想通信下多车协同控制系统研究
近年来,随着通信技术的发展,相关网联化技术给智能汽车突破现有感知、决策、控制等多个环节技术瓶颈带来了更多可能,在安全、高效、舒适与节能等多维度提升了现有智能车性能。然而在处理基于网联技术的应用过程中,现有通信技术的一些固有缺陷或限制不可避免地影响着网联应用的应用范围及表现,例如网络时延、丢包、量化误差、时变拓扑、信道衰减、带宽限制等,某些因素在部分情况下甚至会严重威胁车辆网联应用安全。因此有必要针对非理想的通信因素下车辆控制方法和理论开展研究,为在现有通信限制条件下实现安全可靠高性能的网联车辆控制提供基本的理论基础,为在部分突发通信故障情况下提供降级控制器设计思路,提升智能网联车辆系统安全性。
实验室原有研究考虑了对数量化反馈的马尔科夫线性跳变系统,推导了随机意义下的Riccati不等式,并基于求解线性矩阵不等式的方法求解对应于不同模态的跳变控制参数。基于上述方法,本研究利用矩阵增广的方法描述了包含了有限跳变时延的网络对数量化反馈控制系统,利用上述方法求解不同时延状态下镇定的控制参数,并利用缓存的有限步历史时刻的控制量计算当前时刻镇定的控制量,在实际控制中,根据当前时刻通信时延大小切换不同对应控制参数,以达到镇定系统的目的。通过网联车辆横纵向控制场景仿真测试及和常规控制器控制性能的对比,验证了本研究为跳变时延下的网联车辆控制提供了一种可行的控制器设计思路。
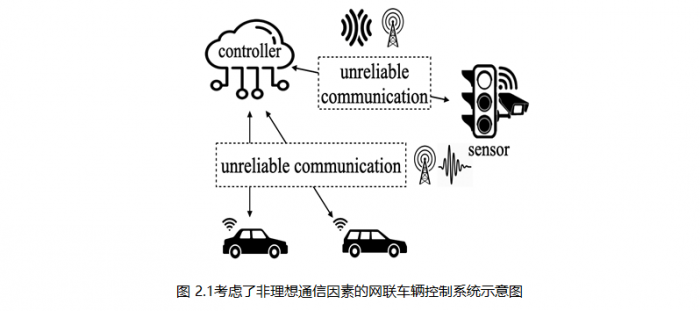
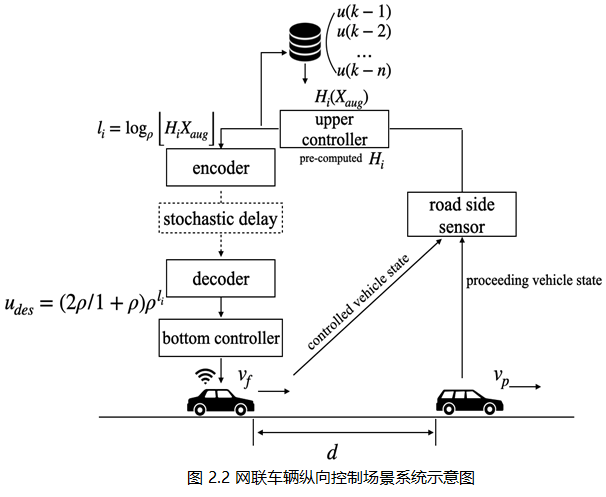
研究骨干:潘济安
- Pan J, Xu Q, Li K, et al. Controller Design for V2X Application Under Unreliable Feedback Channel[C].2019 IEEE Intelligent Transportation Systems Conference (ITSC). IEEE, 2019: 2496-2502.
3. 面向多场景的智能网联车群共性决策与控制方法
传统的智能网联汽车控制方法大多面向单一场景,难以在场景间进行平滑切换,且其研究场景往往过于简化,难以在进行实际应用。因此,研究面向多种复杂交通场景的共性决策与控制方法,对于智能网联交通系统的整体效率和经济性提升具有重要意义。
提出了基于协同指派的智能网联汽车多车协同编队控制方法,采用集中式决策、分布式控制的架构,在带有出、入匝道的多车道高速公路场景下,根据车群、道路参数动态生成多车编队几何构型,构建了车辆-编队内目标位置指派问题模型并选取匈牙利算法进行实时求解,使用分段贝塞尔曲线进行车辆运动轨迹规划与车辆控制,拓宽了现有多车协同控制领域的场景复杂度,提升交通系统整体效率的同时,也提升了车辆的行驶安全性和燃油经济性。
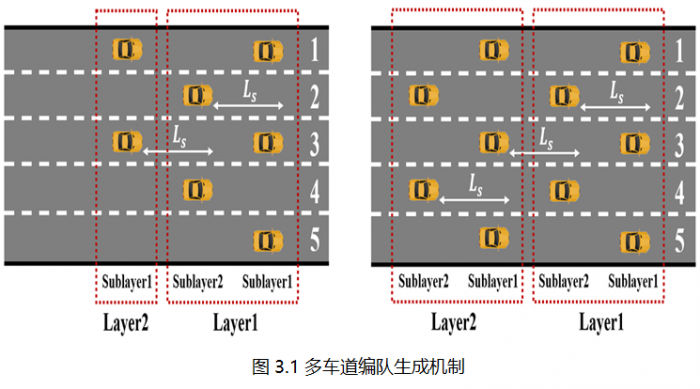
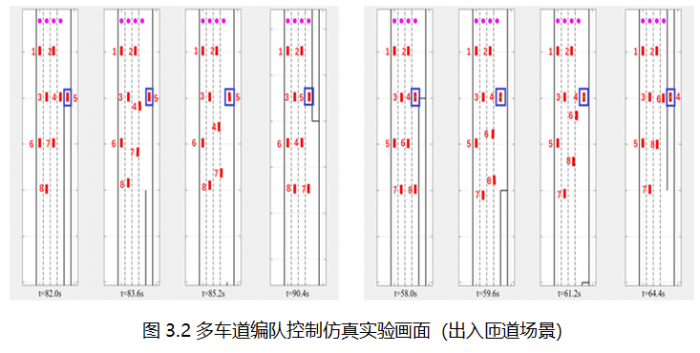
提出了基于车群指派的多车道无信号灯交叉路口通行控制方法,在宏观和微观两个层面分别进行车群通行序列的计算和车辆运动的规划与控制。在宏观层面,构建了多车道交叉路口交通流冲突模型,使用基于图论的树搜索算法计算车群间的无冲突通行序列;在微观层面,提出了车群重组方法,按转向需求对原车群进行拆分和重新组合。本方法解决了现有交叉路口控制方法难以应用到多车道场景的难题,相比于传统方法,提升了交通效率和燃油经济性。
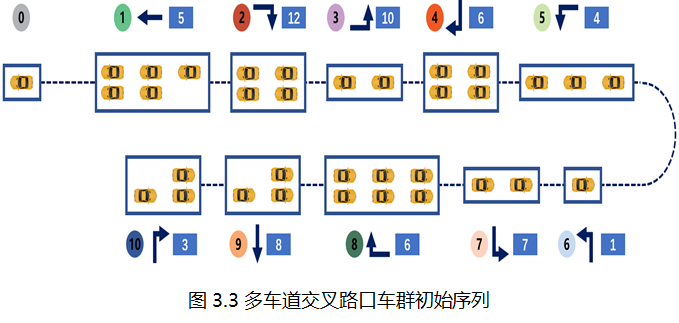
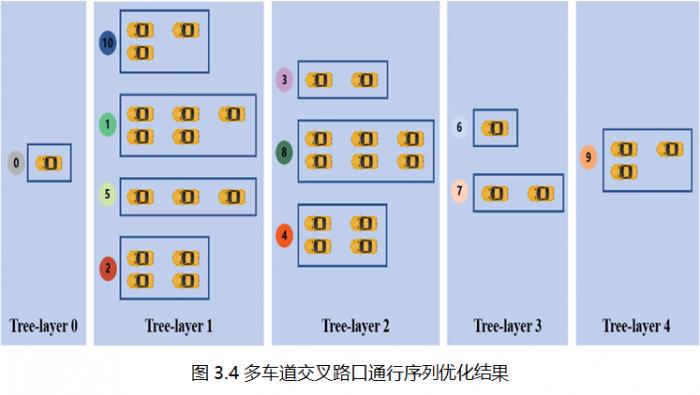
研究骨干:蔡孟池
- Cai M, Xu Q, Li K, et al. Multi-lane Formation Assignment and Control for Connected Vehicles[C]//2019 IEEE Intelligent Vehicles Symposium (IV). IEEE, 2019: 1968-1973.
4. 混合交通系统性能研究与最优控制器设计
传统的智能网联汽车技术多针对所有车辆全部自动驾驶的工况,在实际的智能网联汽车技术推广过程中,势必会存在一个长期的过渡阶段,即智能网联汽车(ICV)与驾驶员驾驶汽车(HDV)共存的混合交通工况。研究两类汽车共存的混合交通系统,设计针对混合交通环境的智能网联汽车技术,对于理解智能网联汽车对交通系统的影响机理、提升过渡阶段ICV对交通系统的积极作用具有重要意义。
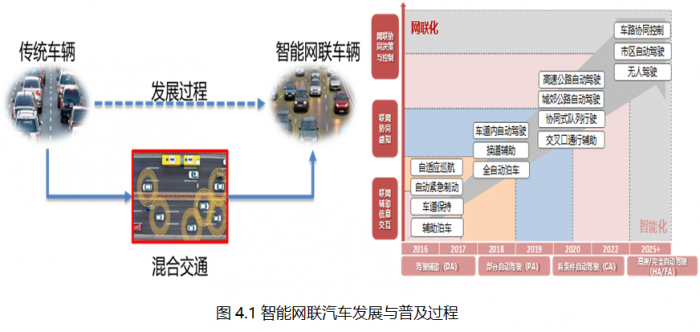
为研究ICV对交通系统的影响机理,研究针对封闭环形混合交通系统,从理论上分析了该系统的稳定性、可控性、可镇定性以及可达性,证明了由一辆ICV和多辆具有匀质/异质动力学特性的驾驶员驾驶汽车构成的环形混合交通系统在一定条件下的可镇定性,求解了通过ICV主动影响提升交通流速度的上界,揭示了ICV在低渗透率下削减交通扰动、提升交通效率的内在因素和发展潜能。
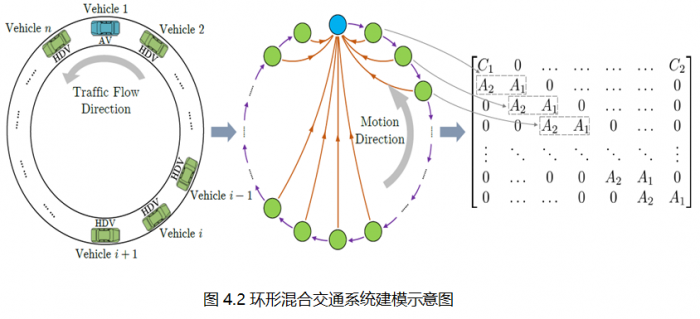
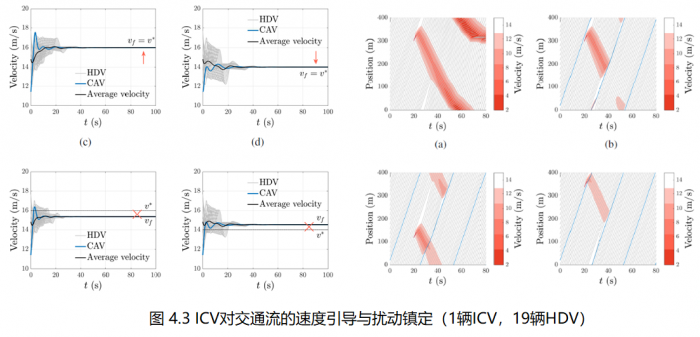
为解决混合交通工况中通信能力受限条件下的ICV控制策略设计问题,研究将其构建为带有结构约束的最优控制器求解问题,并利用稀疏不变性原理构建原问题的凸放松,从而求得次优的ICV控制策略。此外,传统的ACC、CACC等策略着眼于ICV自身的性能,本策略直接通过控制ICV优化整体交通系统,使得ICV在低渗透率下具有显著提升交通性能的效果。
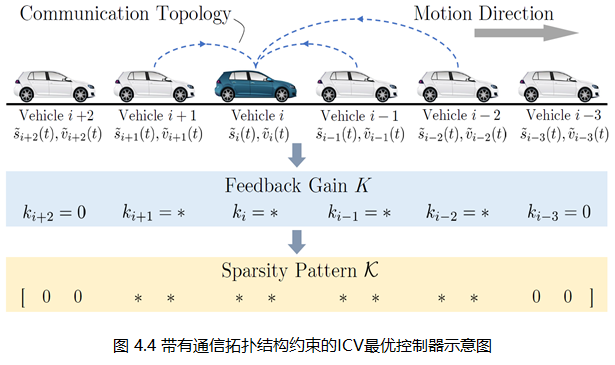
研究骨干:王嘉伟
- Zheng Y, Wang J, Li K. Smoothing traffic flow via control of autonomous vehicles[J]. IEEE Internet of Things Journal, 2020.
- Wang J, Zheng Y, Xu Q, et al. Controllability Analysis and Optimal Controller Synthesis of Mixed Traffic Systems[C]//2019 IEEE Intelligent Vehicles Symposium (IV). IEEE, 2019: 1041-1047.
创建: Mar 05, 2021 | 10:56